Options
Text Feature Extraction and Representation of Chinese Mental Verbs Based on Deep Learning
Journal
Scientific Programming
ISSN
1058-9244
Date Issued
2022-09-08
Author(s)
Yongxue Wang
Editor(s)
Lianhui Li
DOI
10.1155/2022/7496054
Abstract
There are some problems in feature extraction and representation of Chinese mental verbs, such as low accuracy and low efficiency. In order to further improve the computational efficiency and accuracy of Chinese mental verb text, based on deep learning theory, activation function and damage function were used to optimize the original model. Considering the calculation method of model gradient, the optimization model describing the characteristics of Chinese mental verbs is finally obtained. The model can be used to analyze the variation of the characteristic parameters of Chinese verbs and the method of representation. Finally, the model error is analyzed by the method of comparative verification. Relevant studies show that the number of outputs and output results corresponding to softmax function will influence the test results of the model. By comparing the curves, it can be seen that the curve corresponding to the output number has an obvious increasing trend, while the corresponding output result curve has an opposite changing trend. The linear and nonlinear characteristics of the two curves are obvious. The real value of the mean square error function shows a change of linear increase, while the corresponding output value shows a change trend of gradual decline, which indicates that the two kinds of data have different influences on the model under related algorithms. It can be seen from the error data that the gradual increase of independent variables will improve the accuracy of the test results. Five different Chinese mental verb parameters have different manifestations in the deep learning model: among them, declarative verbs fluctuate in a small range and have little corresponding influence. However, the fluctuation of nondeclarative verbs and positive and negative declarative verbs is relatively small, and the curve is relatively stable. Negative verbs have a positive influence on the test output. Double negative verbs have negative effects. Finally, the accuracy of the model is verified by calculating the difference between experimental data and model data. This research can provide theoretical support and model verification method for the application of deep learning model in other fields of Chinese language.
File(s)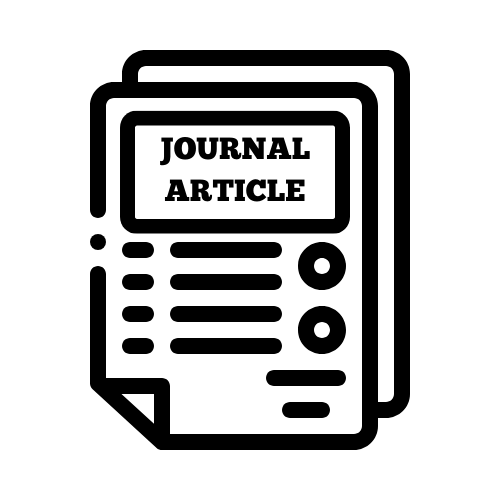
Loading...
Name
Journal Article.png
Size
17.27 KB
Format
PNG
Checksum
(MD5):85f5e85fa8f8c13d7350540217a227b6